As the world rushes headlong into the era of artificial intelligence, the recent acceleration of AI adoption has been sudden, pervasive and eye-opening for organizations and governments alike. Though AI adoption can result in savings of time and effort, negligent implementation can lead to harmful bias, discrimination, and adverse consequences. In the U.S., these outcomes have prompted calls for an AI Bill of Rights and motivated organizations to focus on developing AI governance frameworks to aid in compliance, transparency and responsible use of artificial intelligence technology.
The further down the road of AI your organization goes, the more valuable an AI governance framework will prove, especially in the emerging areas of data lineage, sharing and scoring.
What is an AI governance framework? Why is it necessary?
An AI governance framework is the set of internal organizational structures of roles, responsibilities, and data architecture to ensure trusted outcomes of AI systems.
The aim of any AI governance framework is to make sure that the decisions that stem from AI algorithms do not infringe on the rights and privacy of individuals, involve a harmful bias, and that appropriate transparency and controls are in place to explain and adjust artificial intelligence rationale.
A pressing problem for many organizations is ensuring that they maintain control over their AI models, as most AI models have not had traditional business controls or curation.
For example, if you develop an AI model for, say, predicting fraud or creditworthiness, will your organization be able to stand behind the decisions it makes? If consumers claim gender bias or demographic profiling based on the model’s predictions, can you justify the actions taken as a result?
Key components for any AI governance framework
To most business users, AI is a black box: input is provided and the algorithm returns a result. They may know a little bit about the data on which the software bases its predictions, but not much more.
The goal of an AI governance framework is to help convert the black box into a glass box. When users know and understand the data, it’s easier for them to trust predictions based on that underlying data. Larger clients are approaching AI Best Practices through the creation of AI Center of Excellence to track, measure and monitor their AI programs holistically.
Data quality is paramount. That’s especially true in data pipelines because it’s not good to have bad data flowing from one department’s AI model to another.
For any organization to implement an AI governance framework with success, there are three key components needed to maintain and ensure necessary data quality:
- Data lineage – You can see, down to the column level, how data has been managed and propagated, and whether it’s good or bad.
- Data scoring – Scores take into consideration the results of profiling, along with how users themselves have evaluated and ranked the quality of that data. Scoring summarizes how complete and effective the governance around the data is. Couple this with data drift and the metadata becomes actionable, alerting when data falls outside the set data profiling thresholds.
- Data sharing – Once your organization has identified and highlighted good data, the logical next step is to make it available to other users. Data marketplace tools let users analyze, compare and share governed, high-value datasets and AI models.
AI governance framework considerations
Many companies have jumped into AI with both feet by ramping up staffing and applying AI wherever possible. However, there are some key considerations every organization should address before increasing their usage of artificial intelligence.
Scattered organizational AI efforts
Many companies have widely scattered pockets of AI activity in disparate departments, for example, marketing, engineering and finance. One problem is that these departmental pockets of AI activity have generated lots of code – and maybe rolled out applications – but perhaps without the usual process of collecting input from the business or with appropriate guardrails around data. Smart organizations are putting together in-house centers of excellence to foster safe, profitable AI.
Business-oriented data understanding
It’s worth noting that most AI models are developed by data scientists rather than by the people who work in the business every day. The data scientists may know that relationships in data are important, but they don’t have the years of experience in the business that go into understanding those data relationships. While they know there’s a difference between good and bad data, they don’t have the experience to recognize good data from bad intuitively.
Maintaining on-going institutional knowledge
Many organizations aren’t strangers to employee turnover. In practice, that could mean that a team of people come into your organization, build an AI model and leave a black box. That makes it incumbent on any organization to use tools, processes and systems to store that institutional knowledge, then maintain, sustain and grow it.
Upholding AI safety principles
Suppose your company follows the AI Bill of Rights as an example. Your AI governance framework will dictate that you allow for several principles:
- Protection from faulty or unsafe AI systems
- Protection from discriminatory AI and algorithms
- Privacy and security when dealing with AI
- Notice and explanation when an AI system is being used and how that can affect outcomes
- The right to opt out
You can use automation tools to uphold those principles and reinforce your AI governance framework. The tools make it easier for you to observe changes in your data and strengthen your protections around AI.
From a business perspective, you’ll put roles and responsibilities in place to clearly state what you expect your AI models to produce and monitor what they are producing.
Finally, AI governance will specify the intent of the AI model from a business perspective. After all, nobody wants to – or, nobody should want to – do AI just for the sake of AI. Somebody somewhere in the organization will want to profit from AI and the resulting insights, and an AI governance framework includes a way to curate the models from a business perspective which will include items like data scoring, and answer questions like, “What is the intent of the model?” And, “What insights were achieved?”
Trusted data and model drift
Data scientists are known to spend much more of their time finding and cleaning up data than they spend developing logic. By capturing the definition, structure, lineage and quality of the data, your AI governance framework has the potential to flip that ratio of time spent cleaning data versus utilizing data for insights. It can guide users and analysts to trusted data instead of making them work for it.
But even if your analysts have their hands on trusted data, too many AI projects still fail. Why? Because—as mentioned earlier—the nature of AI models is that they work differently with time. Model or data drift occurs when you train your model based on a given dataset (say, urban consumers), then run the model in a different environment (such as rural consumers). Although anomalies and biases may not be apparent in your initial tests, your AI model continues adapting to the new environment, causing the resulting predictions to vary over time.
In fact, unless you’re deliberately tracking drift, you may not identify it at all. Instead, you may conclude that your project has failed. Since the quality of your predictions depends on what you feed into the model, tracking drift means keeping a constant pulse on observing the movement and context of your data.
Pitfalls and mistakes in AI governance
Not monitoring data and model drift
Without an AI governance framework in place, you can’t monitor your progress adequately. When an AI model goes into production, it usually does what it’s supposed to do. The big difference is that with traditional software the same input always generates same output, whereas an AI model is designed to learn, change and evolve over time. The model could take the same input at different points in time and make different predictions. Every organization working with AI needs to keep that in mind.
Then comes the question “How long will it take us to get AI right?” Your exposure may be low with things like natural language processing; your app may mis-transcribe a recorded conversation. But what if erroneous answers from your chatbots or misidentification from facial recognition reveals bias in your training data? How much customer frustration and tarnished reputation will you endure for the sake of your AI effort?
Even if your AI models work without a hitch, they will eventually start making decisions that you haven’t anticipated. That’s why governance and monitoring are important.
Forgetting that transparency is the goal
The biggest mistake is to realize that your AI effort is a black box and not try to change that. Transparency – meaning structure, observability and understanding of the data – is at the heart of successful AI projects. Unless your governance tools are showing you, for example, how to avoid discriminatory AI and everywhere you’re storing personally identifiable information (PII), you’ll be flying blind.
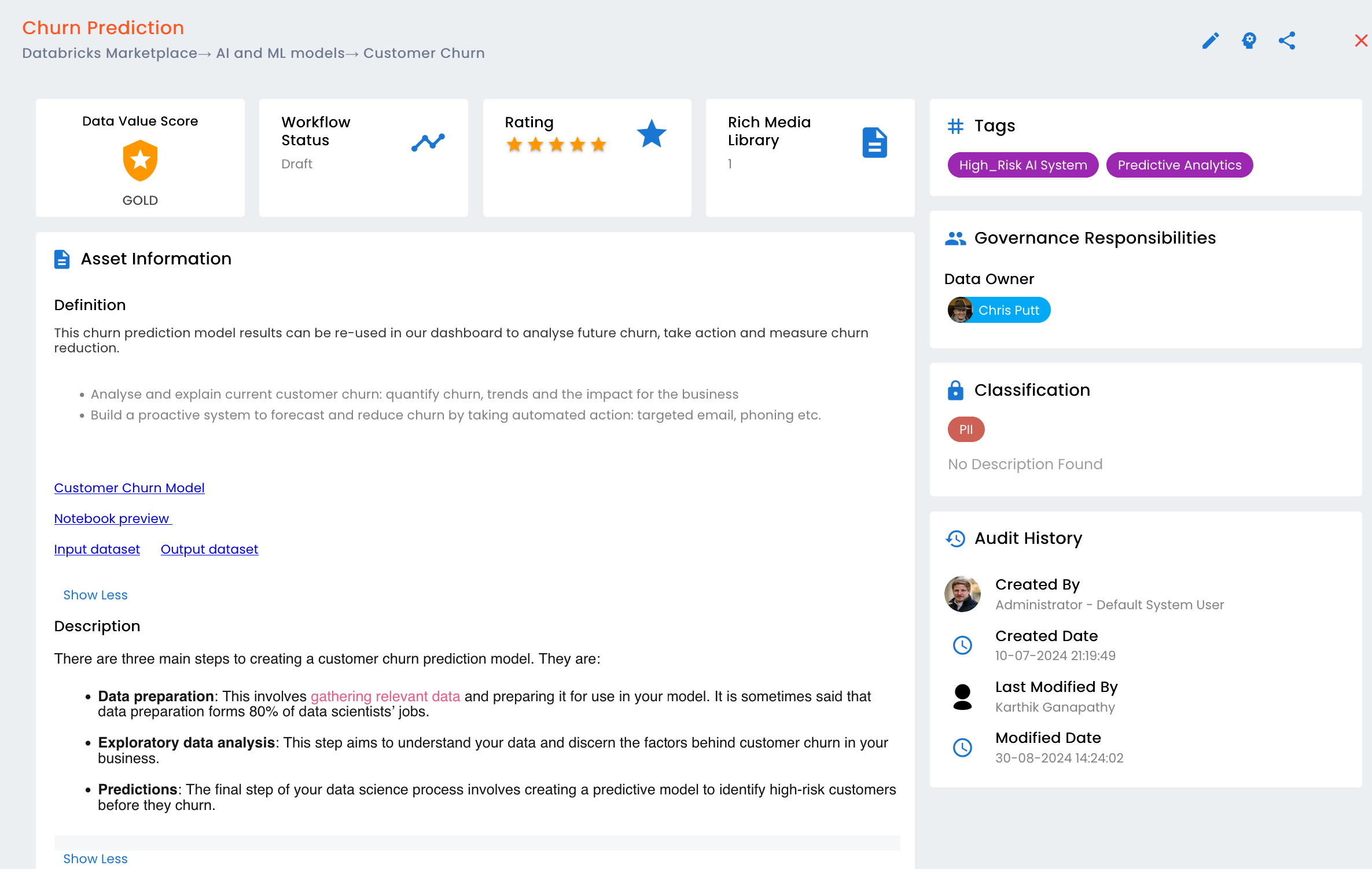
Is your data and governance
AI-ready?
The right model, the wrong problem
Companies often try to use the right model to solve the wrong problem. Suppose you develop a sales forecasting model. Once you roll it out worldwide, it generates accurate predictions on North American sales but offers woefully inaccurate predictions about East Asian sales. A difference like that is most probably due to the data on which the model was trained; here, it was likely trained on North American data. To get accurate predictions elsewhere would require region-specific training data.
For most companies, the biggest mistakes boil down to overlooking the fact that it’s not the model that’s the core component; the data is the core component. Because data changes over time, your organization should not be surprised when models provide unexpected results. Monitoring allows you to control that.
Conclusion
AI models are not one-and-done. You cannot expect that the AI model you deployed in the last sales cycle or economic cycle will keep doing the same thing you expect it to do. AI requires constant monitoring and adjustment as data evolves.
Your AI governance framework helps provide transparency into the fact that you always know what your AI models are doing, introduces the voice of the business to AI development and drives positive business outcomes. An AI Center of Excellence will create focus, immediacy and develop best practices for the organization to achieve the most accurate results possible. It allows you to get more people involved, thereby converting the black box into the glass box through a transparent structure and platform for safe, profitable AI.