Only 33% of enterprises currently have the data quality management (DQM) processes they need to ensure the trustworthiness of their data, according to a 2024 report from TDWI Research. Pause and think about that for a second… two out of every three organizations don’t really know if they are making business decisions based on good or bad data.
To provide some insights on how to improve your data quality management processes, this article serves as a primer for planning the evolution of your data quality management initiatives, their importance, the challenges you’ll face and best practices for strategically improving how you manage data quality going forward.
What is data quality management and why is it important?
Data quality management is a set of practices, processes, policies and standards for managing and ensuring the accuracy, consistency, completeness and relevance of data throughout an organization. It is essential for efficiently and cost-effectively delivering reliable and trusted data to users, enabling sound business decisions, facilitating personalized customer experiences, meeting compliance obligations and supporting AI-driven initiatives while minimizing risks from incorrect or incomplete information.
The six pillars of data quality
There are six pillars, or dimensions, that enable you to assess and measure the quality of your data and the impact your data quality management program is having over time. Let’s take a cursory assessment of how each applies to your data.
- Accuracy: How precisely does your data represent real-world information without errors?
- Completeness: Can you access all the data you need to yield meaningful insights and decisions, or do you have gaps in your records that need to be addressed?
- Consistency: Do you enable seamless cross-system integration and analysis with data fields that are uniformly formatted across datasets?
- Timeliness: Is your data up to date and available when needed for analysis and decision-making?
- Validity: Does your data adhere to the required formats and constraints (e.g., data type, range) for each data point?
- Relevance: Is your data and its relationship to the business well understood and in alignment with your organization’s business rules?
The benefits of a strong data quality management program
A data quality management program is foundational to ensuring your organization is operating with good data. Here’s an overview of the benefits your organization can realize:
Enhanced decision-making
Reliable, trusted data provides accurate insights, leading to better business decisions, risk mitigated AI applications and other data-driven innovation.
Increased operational efficiency
A focused data quality management program can improve productivity across teams by reducing errors, rework, manual corrections and redundant tasks.
Improved customer satisfaction
You can enhance customer interactions by providing accurate, timely and trusted insights for personalized experiences.
Compliance assurance
A data quality management program can help your organization meet its regulatory requirements, such as GDPR or HIPAA, by maintaining consistent and accurate data records.
Cost savings
It prevents costly errors from poor data, lowers storage and processing costs by reducing duplicates, and avoids penalties related to compliance breaches.
The biggest challenges in data quality management
While a data quality management program will yield some compelling benefits to your organization, there can also be some serious challenges to its implementation, evolution and maintenance. Here’s some of the more significant hurdles:
- Data silos: When you have fragmented data across multiple systems, it can lead to inconsistencies, and it becomes quite a bit more complex to achieve a unified view.
- Data volume and velocity: Ever-increasing amounts of data flowing from various sources in real-time can strain your resources for data cleansing and validation.
- Lack of standardization: Datasets with inconsistent data formats and naming conventions will hinder integration and analysis.
- Limited resources: Any constraints that affect your budget or staffing will likely impact your ability to monitor and maintain data quality.
- Compliance and security concerns: Meeting stringent regulations and safeguarding data integrity throughout the lifecycles of your data can be a complex and resource-intensive undertaking.
Best practices for improving data quality management
Your organization depends on effective data quality management practices to ensure your data stays trustworthy, relevant and consistent. To mature your efforts and align with the goals of your business, consider the following recommendations.
Establish data standards and governance policies
Leverage data models and active metadata catalogs to infer, create and implement rules and guidelines for data entry, formatting and validation to ensure consistency.
Monitor and profile data
Continuously monitor and address all data quality issues.
1. Data accuracy
- Completeness: Check for missing values in mandatory fields.
- Correctness: Validate data against known benchmarks, rules or authoritative sources.
- Precision: Ensure numerical data matches the required level of granularity (e.g., decimal places)
2. Data consistency
- Format compliance: Validate data formats (e.g., date fields: YYYY-MM-DD).
- Standardization: Monitor adherence to standard naming conventions or taxonomies.
- Referential integrity: Ensure relationships between tables or datasets are intact (e.g., foreign keys match).
3. Data timeliness
- Currency: Ensure data reflects the most recent updates or changes.
- Latency: Monitor delays between data capture, storage and availability.
4. Data integrity
- Duplication: Identify and resolve duplicate entries.
- Record linkage: Verify unique identifiers for merging or correlating datasets.
- Traceability: Confirm data lineage and audit trail completeness.
5. Data completeness
- Coverage: Check whether all required datasets or sources are included.
- Mandatory fields: Ensure no critical fields are left blank or null.
6. Data conformity
- Business rules compliance: Validate data against predefined business logic.
- Code validity: Ensure coded values (e.g., country codes) are within accepted ranges.
7. Data uniqueness
- Primary key validation: Check for duplicate primary key violations.
- Entity resolution: Confirm that entities (e.g., customer IDs) are uniquely identifiable.
8. Statistical monitoring
- Outliers: Identify values that deviate significantly from statistical norms.
- Distribution analysis: Check for unexpected shifts in data distribution patterns.
9. Data usability
- Readability: Ensure data is presented in a user-friendly format.
- Relevance: Validate that data meets user needs for specific use cases.
10. Metadata and documentation
- Schema drift: Monitor changes in data schemas over time.
- Data definitions: Ensure fields are clearly defined and documented.
11. Context-specific metrics
- Industry standards: Align with domain-specific quality measures (e.g., HL7 in healthcare).
- Custom rules: Profile data against organization-specific quality rules or KPIs.
Invest in data cleansing and enrichment
Automate remediation processes to regularly clean and update your data, removing duplicates, correcting errors and adding missing information.
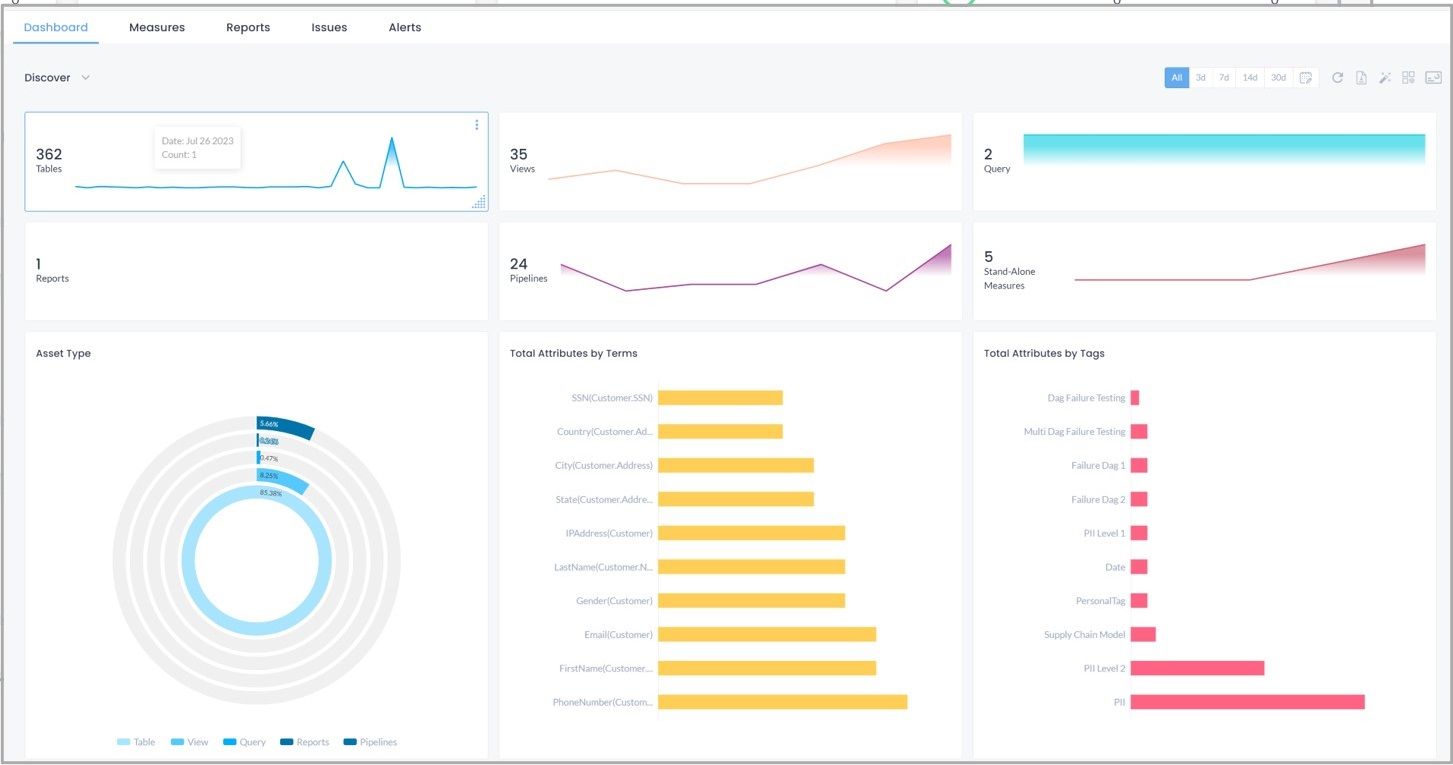
Do you trust your data?
Implement data ownership and accountability
Designate data stewards for different departments to oversee data quality and address any issues.
Publish data quality scores within your data catalog and beyond
Analyze and score the quality of your data and combine it with other health and lineage information to provide quality metrics and data value scoring in order to increase stakeholder and end user trust in the data.
Continuous training and collaboration
Educate your teams on data quality standards and best practices and establish stakeholder feedback loops to foster a culture of quality management across the organization.
Conclusion
To succeed in today’s data-driven landscape, your organization needs to achieve and maintain quality and trustworthy data. Despite the challenges, implementing robust data quality management practices will ensure your decision-making processes are based on reliable, accurate and relevant data. By focusing on best practices—such as establishing governance policies, leveraging advanced solutions, and fostering accountability—your business can overcome obstacles like data silos, resource constraints and compliance demands. And as you advance your DQM initiatives, you will position your organization for improved decision-making, operational efficiency and customer satisfaction while mitigating risks and cutting costs. The journey to better data quality is not without hurdles, but the benefits far outweigh the effort.