In the pursuit of an AI governance strategy, there is a wide range of organizations who tether between cumulating their data and AI projects in one area of the business to those who are trying to get in front of their Gen AI requests, to those building a cohesive strategy to execute a solid foundation of AI-ready data across their organization.
At this point, it’s rare, that organizations have a well-tuned AI factory of classifying, profiling and certifying AI data, and producing AI insights that are advancing — let alone, disrupting the business. But the intent to put their data house in order and define their AI strategy is in motion for the majority of the data leaders I meet with weekly. At the end of the day, AI offers a creative new world of endless possibilities, and we have the opportunity to shape that picture versus letting AI shape us.
Organizations who have already implemented a data catalog of some sort are ahead of the AI train for sure. Their data has been modeled, classified, curated and diagrammed. Catalog vendors are racing to the forefront with many new capabilities that go beyond governing data but make no mistake, these core capabilities are the main ingredients to a well-baked cake sure to delight your AI insights and outcomes. New catalog use cases like observing data pipelines, scoring data, monetizing and marketplace capabilities are creating savvy data communities and data leaders who can focus on taking next steps with the AI results and outcomes versus data clean up and mammoth migration efforts. At the end of the day, data performs best on well-defined, accurate, structured data.
When it comes to building a strategy to prepare your data for AI use, below are seven best practices to apply.
1. Pre-define the outcomes and characteristics of your new AI deliverable
It’s important to be specific about the intent of your new AI deliverable — outline questions and expectations of what answers you are trying to achieve. In a sense, you want to become a “prompt specialist” learning new ways and methods of asking defining questions that narrow down the subject area to align with the goal of the AI model. This way, as you observe the data, the outcome you are trying to achieve will be monitored and tuned as you accept or reject data anomalies.
Leverage data modeling to outline the data requirements for any new AI use cases. This is where a new “Data Product” comes into play. When business consumers come to the data engineers and ask, “I need data for this new marketing campaign, new product, new study,” etc., they are asking for every data asset available to help them with their new initiative. This could be existing datasets, reports, and AI features, coupled with any guardrails (policies and guidelines) they need to be aware of when using said data. Data products achieve one very important ingredient to success— combining business consumers with data experts to have a fruitful experience and resonate outcomes.
2. Self-serve data to your analytical community
Searching and requesting analytical data shouldn’t require an IT work order. A solid data catalog marketplace should be a fun collaborative experience with easy methods to bring data to life for any data consumer. Consider providing an Amazon-like shopping experience where end users can compare and uncover related datasets, create a new data product with multiple datasets, and collaborate on data scoring and issue threads. This becomes governing data without knowing it.
You don’t always need gold star data when you are building AI, because you will run out of data. But you will always need transparency into the data characteristics. Especially when you may be using synthetic data, you need to know that this data was created internally. Where did the data come from? How often is this data used? How well liked is the data? Has this data been profiled? If so, did it meet the level of integrity that we accept as an organization?
Maybe It’s ok to use below average data to produce a more well-rounded insight. Maybe you need to be precise and fully accurate because there is regulatory information at stake. Identify the qualities your data must meet and the parameters for how data will be used in each context.
The analytic marketplace request in itself has mandatory attributes for defining what business initiative is this currently being used for, what are the expected outcomes and insights, and what area of the business will monitor the data pipelines for this model, etc. It should be your gateway to internal, external, and third-party data that has been classified, curated and governed.
3. Classify your data landscape
Most LLM’s and AI models need to be pointed at a sub-section or domain of data pertaining to what insights are being produced. If you’re pointing your LLM’s and models at the entire universe of data, it is less likely to find data patterns and insights that pertain to your model. Your entire model is at risk of ever making it to production.
A data catalog will categorize the data for you so that you can point your AI model at customer, product and/or sales data for example. A governed catalog will give you more information like quality characteristics, how well liked and used the data is, and what previous results of using this data were.
If you’re trying to break down your AI models and LLM’s into smaller more usable sections a highly classified data catalog will break the data down for you. This accelerates your search and takes the guess work out of finding the relevant data to feed your model.
If you’re compartmentalizing the data behind AI, you’ll be impressed with using data pipelines to manage and monitor your data inputs and outputs. AI pipelines offer a transparent and actionable view into exactly what’s happening to your AI model in near real time. Visualizations of the data flow highlighted with personal information, transformation rules, and data quality profiling results become a quick map of what’s entailed in producing the outputs of the model. Data quality engines monitor these pipelines regularly for data drift, data anomalies, sensitive data and data degradation.
Another key to classifying data is semantic augmentation. Using semantic augmentation within your data profiling capabilities automates the process of creating business names as you profile your data. When the data quality module is based in your data catalog you have already have the business assets there to perform this augmentation. This becomes a method of tagging your critical data elements, personal information and regulatory data from your already governed catalog. Combine data profiling results, governance, data usage and end user rankings to classify data into gold, silver and bronze tiers that represent the relative value of the data.
4. Develop a strong AI governance framework
The current AI governance regulations worldwide have rapidly become a global focal point, reflecting a complex balance between innovation, ethical considerations, public safety, and competitive economic interests. In response, organizations must establish internal practices and policies that meet diverse, and sometimes conflicting, regulatory requirements. Now more than ever, it is imperative to have a strong AI governance framework in place.
Companies need to ensure that their AI governance framework is ready to:
- Respond to complex and evolving AI policies and requirements
- Detect algorithmic fairness and bias
- Ensure ethical and transparent AI practice
- Develop a flexible and agile technology focused on adaptability and future regulation
- Participate in industry collaborations, such as the Global Partnership on AI (GPAI)
The framework should be the basis for ethics and risk. Be intentional with the reason you are doing AI to begin with, not just because it’s cool. Ethics are tied to the culture of the organization and your AI strategy needs to be informed and transparent when it comes to ethics. This develops into how individuals will go about programing their AI and selecting the type of data to include. Observing data and alerts can become your basis to act on data that has changed. Therefore, be sure to fact check to keep the model on its true purpose and intent. Many times, ethics can be the means to separate fact from fiction.
At the end of the day, reducing bias is hard, because humans can’t spot their own biases or even know where to look. As Pulitzer Prize-winning behavioral economist Daniel Kahneman states in Noise: A Flaw in Human Judgment, “Behavioral economics shines a light on bias blind spots. Uncovering bias is one of the most interesting questions facing the use of AI because it takes social science and computer science to solve it.”
With data changing so rapidly and so many pockets of the organization asking to self-service their data for AI, it is the AI framework that must be in place with the technologies they are using.
Being prescriptive from a risk perspective requires that you identify and disclose the level of risk your new AI model or LLM could pose to your organization. Is it a low, medium or high-risk program? For example, we see customers needing quick automation routines performing data validation on particular tables and views. Which means scanning through tables to identify critical, sensitive data and excluding it from the AI inputs. Or maybe choosing to keep the sensitive data in but tagging it as sensitive data and including the actual policy and business rules of how to use this data.
These are all fundamental functions that a catalog should support, and many catalogs are investing in further capabilities to become more proactive and personalized with alerts and prompts so not to be missed or bypassed by a human.
You want to define and enforce policies on data management, access control, and usage that will align with coming AI regulations and promote responsible AI use. When a data and AI team can ensure data sources are trustworthy, data is secure, and the company is using consistent metrics and definitions for high-level reporting, it gives data consumers the confidence to freely access and leverage data within that framework.
5. Observe AI models to eliminate bias
Design and regularly observe the data feeding your models to easily identify anomalies and missed data integrity thresholds that lead to biases, ensuring the data used for AI is representative, ethical, and free of skewed perspectives. This needs to be done in near real time. Catalogs that have built in data quality components are best at this. You may have a data quality solution that profiles data bit by bit but if you don’t have the catalog giving you the whole picture you will only be monitoring what the data quality tool knows about. And if you have a catalog without data quality, you won’t have transparency into where good, bad, and unmeasured data is propagating or leading you directly back to the true source of that dirty data.
Data changes often, it’s fluid. When you set data thresholds the system should automatically alert you to data that goes above or under the min or max value you have set for that data. When you see spikes in the data, you will be able to accept or reject those spikes. In essence — you are training the data behind your AI model. Maybe you are observing pricing behind a new product. If the price is spiking in one area due to a recent event (hurricane, pandemic, etc.), you may or may not want your AI model to consider this anomaly. If it takes a good three plus months to understand there is a pattern to the data your AI model is producing, you may want to reject anything other than business as usual. On the flip side, letting your data go without observing and training can lead to so many spikes that the model renders itself useless, with no clear insights or learnings.
Modern data quality solutions offer business dashboards and views. Business definitions and rules will be considered in the profiling of the data. Combine this with the catalog and you have a one-stop marketplace to trace, track and deliver data that is trusted for any analytical program or project.
6. Score and certify your AI
Part of bringing the organization along with you on the AI journey is including your data consumers with roles and actions. Maybe there’s a data maturity model that outlines what steps you need to take to verify that you are using trusted data. Determine what needs to be in place for that AI model to hit production before it’s released. Tie this to your new AI models and you have an AI certification process. This should help you sleep at night.
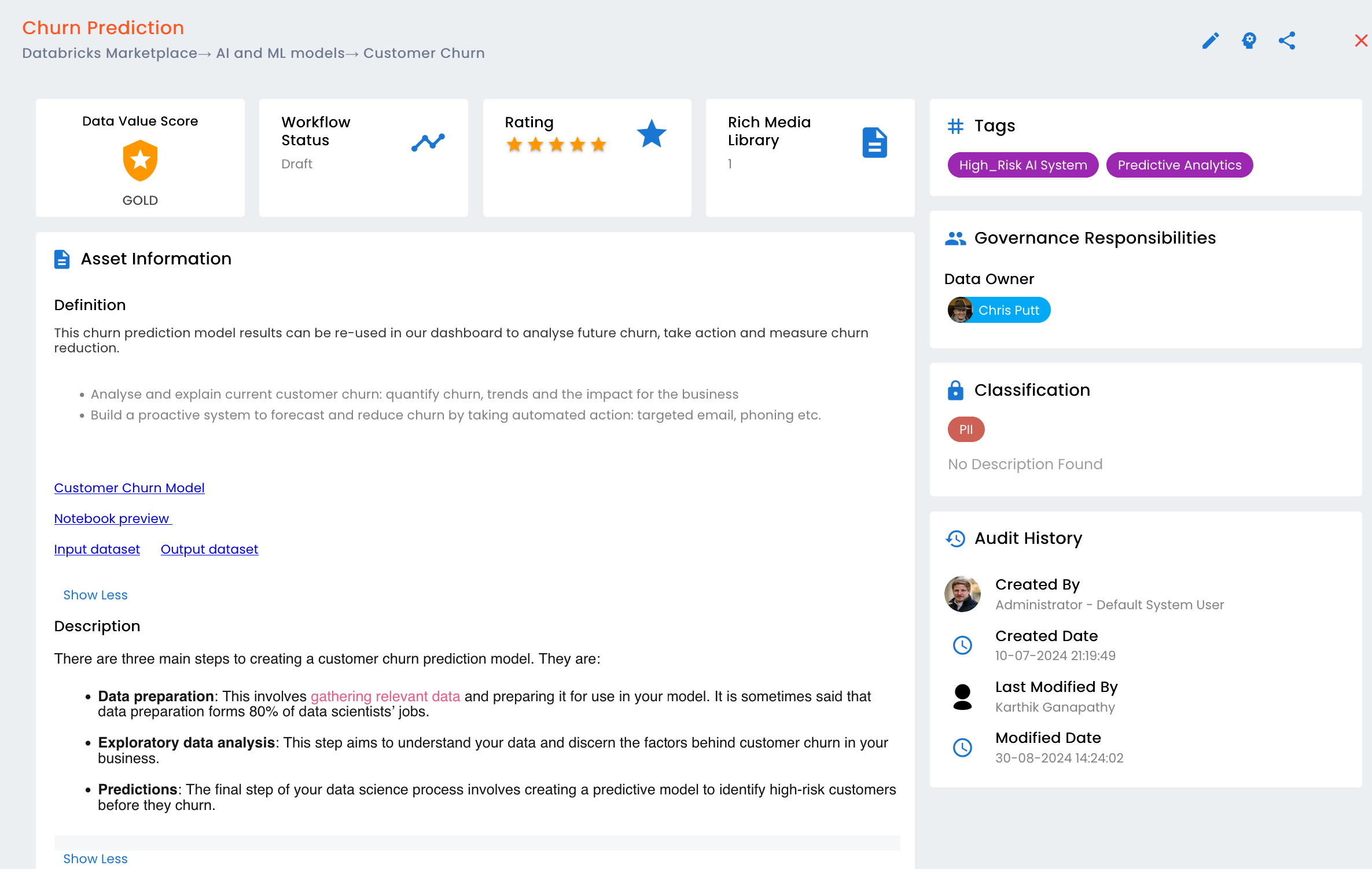
Is your data and governance
AI-ready?
Data scoring typically includes end user ratings, data quality profiling results and end user rankings. These are typically the facets that classify your catalog data into gold, silver, and bronze datasets or products. I’m not saying don’t use the bronze data but know the risk and why it has not yet been classified as gold data. This also allows you to see where you are vulnerable with data that has not been classified or profiled.
No matter the strategy, think big, start small, scale quickly.
7. AI training
Don’t forget about training your data and user community on AI itself. Without training, issues and exposures are just brewing. Training users on prompt engineering, AI risk and regulations, and data literacy will eliminate fear and unite the organization on this next new horizon of AI.
Conclusion
The radical transformation needed of data management capabilities has brought about the urgent call for transparent data. Data transparency and a trusted place (i.e.: internal marketplace) for your D&A users to go to self-serve all their data needs is what will equip your organization to be “data ready.” A data model, metadata catalog, data quality tools, and a business glossary are all great starters to get your data house in order, but when it comes to applying these capabilities, you need a portion of each one working together with AI to give you end-to-end use case. Getting in front of the AI train will take realism that starts once again with marrying context to data and managing that context throughout the AI lifecycle.