One of the biggest questions and obstacles when it comes to decision making in any organization is: “Can we trust the quality of our data?” No organization wants to make strategic decisions based on flawed data, and the uncertainty around the quality and reliability of data can be a barrier to revenue and growth.
In this post, we will outline several data quality best practices that will help to improve organizational confidence in the quality of your data and streamline your decision-making process.
What is data quality?
Data quality is often defined as a measure of how accurate, complete, consistent, and relevant data is for an intended purpose.
While “data quality” exists in the data management context, it also has important nuances in the business context.
Naturally, some of the fundamental questions about data quality are “Is the data accurate?” and “Does it contain bad records?” You can extend those to questions like “Is the data complete?” and “Did we miss important information in collecting the data?” You want to know whether the data is missing things that it should contain. That’s where consistency plays a role in data quality best practices.
For example, when you’re creating a form to collect data, you make decisions about which fields will be required and which will be optional. If some of your respondents fill in the optional fields and some leave them blank, can your decision makers consider all the resulting records to be consistent? Some will; others will complain that the data is incomplete. That difference in consistency matters to data quality.
Another element of data quality is business relevance, which can be even more subjective. Are you collecting (and paying to store) data with no relevance to the business purpose at hand? Quality is about more than what is stored in the database; it’s about data being fit for use and supporting the needs of the business. Is what’s supposed to be in the data, actually in the data?
Why is it important to maintain data quality?
Your data is an asset and you rely on it to run your business. You want to get the most out of your data by analyzing it for answers to deeper business questions. What do you want to get out of your data as an organization?
Your focus informs the definition of data quality in your organization. At the most superficial level, you need basic, transactional data quality so you can ship product and get paid. But suppose you want to work at a deeper level and use the data to optimize your customers’ experience. Suppose you want to use the data to find adjacent markets, then grow your revenue and your company. Those goals will expand your definition of data quality.
The profusion of products for running analytics on data is another argument for maintaining data quality. These capabilities are no longer the in the sole domain of large enterprises with deep pockets. It has never been easier for small and medium-sized businesses to analyze and use their data without a dedicated team of number-crunchers. That brings new insights within closer reach of decision makers but makes it more important to base those decisions on high-quality data.
Data quality best practices
1. Involve the C-suite.
If you think you’re going to convince everyone of the importance of data quality when it’s not coming from the folks at the top, you’re probably fighting an uphill battle.
Your C-suite has to believe in data quality because they have to promote and actively participate in a data-first culture. Of course, as the ultimate decision makers for your organization, the C-suite has a vested interest in basing those decisions on high-quality data.
2. Include data quality as part of a data governance framework
For a long time, data governance was limited to policing the way users worked with and applied the organization’s data. Mostly, it was an exercise in telling them what they were not permitted to do with the data. More recently, it has become recognized as getting the most out of your data with the least risk, with users examining and taking responsibility for the upside of data.
That’s why data quality has emerged as a core element of data governance and empowerment, and as one of the main drivers for funding data governance. A basic expectation of a data governance program is that users will be able to demonstrate improved results from being data-driven, which, in turn, depends on data quality.
Your data governance framework covers multiple aspects of any given data asset:
- What does it mean?
- How does it relate to the business?
- Who is ultimately responsible for this data?
- What technology is it sitting on?
- How do users access it?
- What is the level of quality in that data source?
All of those aspects contribute to the fitness-for-use analysis.
3. Include data quality in the responsibilities of data stewards and data owners
If you own and benefit from using the data, then you have a responsibility to ensure its quality as well.
For example, the owner of credit-card transaction data in your organization should be tasked with ensuring PCI compliance. The owner of marketing data should be responsible for CAN-SPAM compliance, and for ensuring that bogus email addresses are combed out.
Most organizations, of course, are not large enough to hire dedicated data stewards and data owners. Generally, the steward or owner of transaction data is a finance officer, and for marketing data a marketing officer; stewardship is a responsibility tacked onto a day job. Even so, the organization should ensure that the responsibility for data quality is part of somebody’s job description.
4. Use a business glossary
You want business users to be able to see data-related terms expressed in their own language. In the same way that data quality at the physical level means more than complete records and required fields, terms in the business glossary mean more than a database schema. Glossary terms apply to business rules around your data that cut to the requirements that the business imposes on that data, which speaks to the metrics of data quality.
A given term in your business glossary could be attached to 10 different fields in 10 different databases. That’s when you begin to see the overarching governance of quality from a business perspective, rather than from a mechanical perspective. It ticks the consistency box on your data quality scorecard because that term is associated with the same metrics and expectations for all 10 of those databases.
Using a business glossary is part of establishing a business perspective rather than technical one, with a holistic view of data quality. It will allow you to understand and consistently assess data quality across your organization.
5. Establish quality metrics
This brings you back to the difference between the mechanical and business perspectives. Mechanically, you assess data quality with data management tools that churn through database records and address problems. But the quality metrics that the business cares about are general and directional: What’s the quality trend in this data source? Is quality improving or declining? Is it consistent? Metrics let you analyze data quality and make decisions such as:
- Putting more controls on a given input
- Adding processes that validate the data and fixing it if necessary
- Waiting until someone raises a trouble ticket on the data
Establishing metrics really comes from defining data quality for that specific aspect. For example, you don’t know what to measure around lead quality until you’ve defined lead quality.
6. Monitor based on those metrics and conduct regular audits
Create dashboards that reveal business intelligence to show how data quality has changed since last quarter and whether targets for quality have been reached. The process is like that for any other business function, but you also want to derive insights that will drive action in your organization.
There is a sustainability element in that the best data quality solution addresses quality before the data’s been created. Enterprise-caliber database platforms allow you to configure specific rules on what can and cannot go into a field and a record. Controlling the input of data before it goes into the database is more sustainable than cleaning up afterwards.
Data quality audits show your progress against metrics. What’s happening with the level of quality in your data? Do you have the right rules? Have they been updated? When the business changed the definition of a particular term, did it notify all affected users? Was the change accounted for in the audit procedure?
If your audit reveals a data quality problem, the best way to solve it is to perform root cause analysis and then address the problem programmatically.
Finally, put procedures in place that allow stakeholders to close the loop and improve data quality. People who work with data will always find quality problems in it, so make it easy for them to get to the person who can solve those problems. If organizational or hierarchical barriers prevent users from easily reporting problems, inertia will win out, the problems will remain and the level of confidence in the data will — understandably — decline.
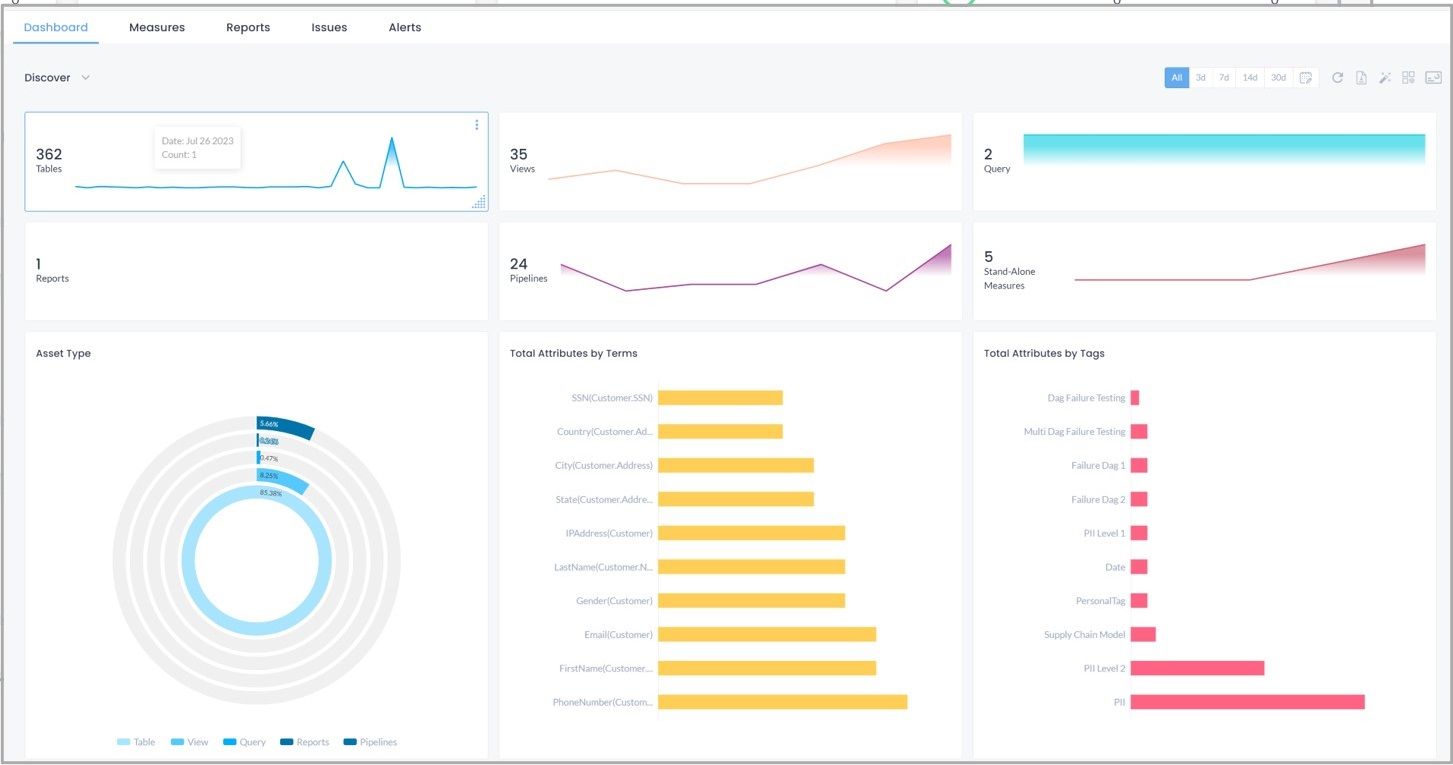
Do you trust your data?
Mistakes organizations make when it comes to data quality
One big mistake organizations make when it comes to data quality is making it solely a function of IT. Why? Because IT doesn’t hold the complete picture in terms of what data quality is. For example, a marketing team will often have select parameters set up to determine if a potential lead should be passed over to a sales team. The data collected from potential prospects may appear complete, but is rife with personal and fake emails that have proven to be an indicator of lack of real intent. This breach in what marketing defines as quality data is not under the IT team’s purview, and would not be identified through normal IT quality processes. With this feedback from the business, processes can be upgraded to remediate this problem and other users can be made aware of the business nature of the deficiencies to assist their fit for use analysis of the data. is not, and should not be alone in the business of data quality.
Another big mistake is not to monitor data quality. That’s like not monitoring air quality or water quality. Where will that get you?
Some companies look at data quality from a very narrow or tactical perspective, figuring that there will always be quality problems and it’s somebody’s job to fix them. It’s necessary to open the organization’s eyes to the impact of data, which will then open their eyes to the impact of poor-quality data.
Conclusion
Data quality is a function of organizational culture. As mentioned above, one big reason that people don’t get what they need out of data is that they don’t trust the data. The biggest reason for lack of trust is having been burned by poor data quality previously. That’s why a culture of data quality is integral to a data-driven, data-first culture. You can’t embed it in your organization as an afterthought; if competitive advantage and growth are important to you, then it’s crucial to create a culture that prioritizes data quality.